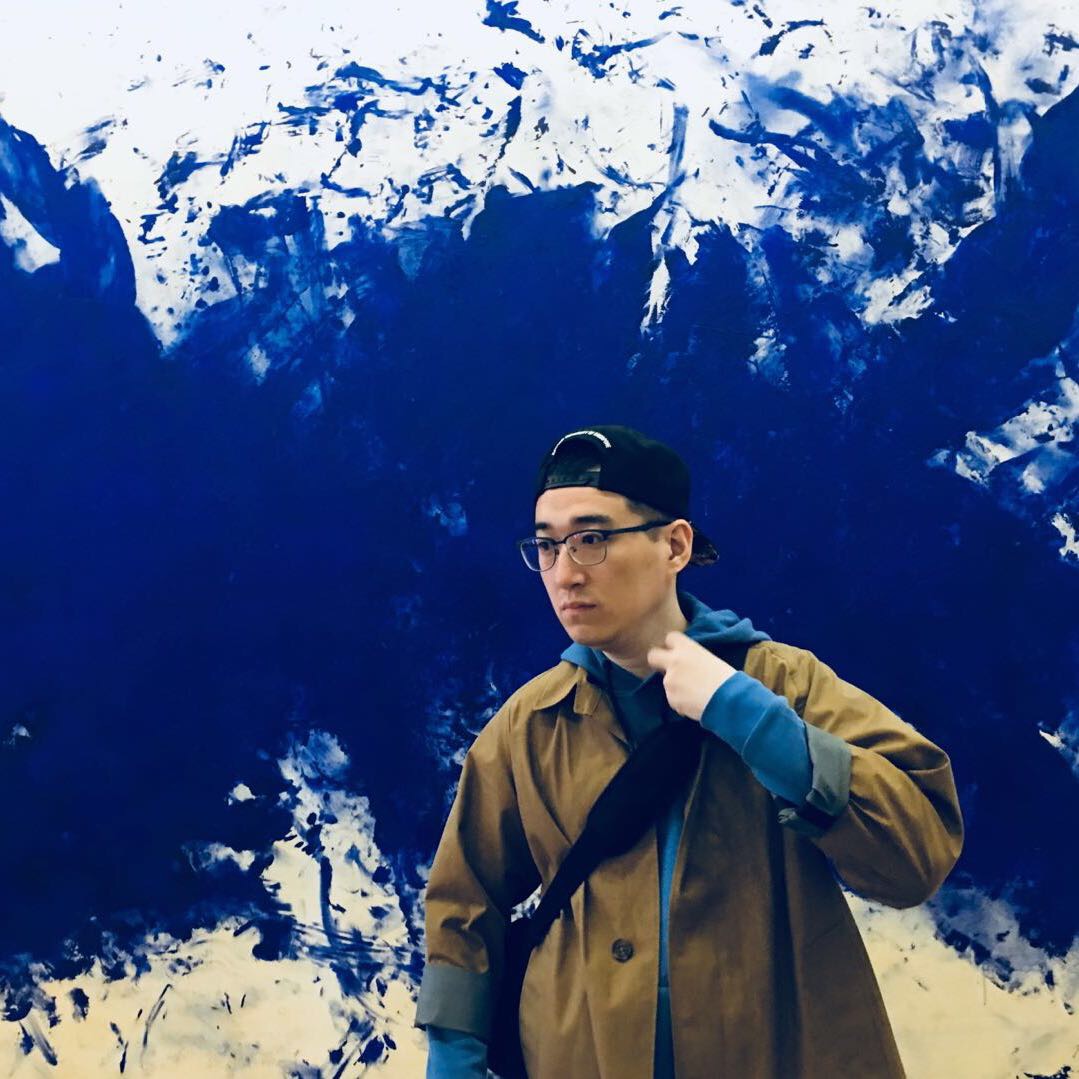
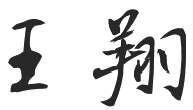
Professor
School of Data Science
School of Cyber Science and Technology
School of Information Science and Technology
University of Science and Technology of China
Email: xiangwang1223 at gmail.com
• Google Scholar Page • GitHub Page
Biography
I am a Professor in University of Science and Technology of China, where I am a member of Lab of Data Science. With my colleagues, students, and collaborators, we strive to develop trustworthy deep learning and artificial intelligence algorithms with better interpretability, generalization, and robustness. Our research is motivated by, and contributes to, graph-structured applications in information retrieval (e.g., personalized recommendation), data mining (e.g., graph pre-training), security (e.g., fraud detection in fintech, information security in system), and multimedia (e.g., video question answering). Our work has over 50 publications in top-tier conferences and journals. Over 10 papers have been featured in the most cited and influential list (e.g., KDD 2019, SIGIR 2019, SIGIR 2020, SIGIR 2021) and best paper finalist (e.g., WWW 2021, CVPR 2022). Moreover, I have served as the PC member for top-tier conferences including NeurIPS, ICLR, SIGIR and KDD, and the invited reviewer for prestigious journals including JMLR, TKDE, and TOIS.
Prospective Ph.D., Master, and Undergraduate Students
I am looking for highly motivated students (PhD, master, undergraduate students) to work together on trustworthy deep learning on graph, especially pre-training, interpretability, generalization, and robustness, and their applications in real-world scenarios. Please feel free to send me your CV and transcripts, if you have interest. We are also actively looking for opportunities in research, partnership and commercialization in exciting data science projects.
News
On the Effectiveness of Sampled Softmax Loss for Item Recommendation.
MultiCBR: Multi-view Contrastive Learning for Bundle Recommendation.
On Generative Agents for Recommendation.
Llara: Aligning Large Language Models with Sequential Recommenders.
Let Me Do It For You: Towards LLM Empowered Recommendation via Tool Learning.
General Debiasing for Graph-based Collaborative Filtering via Adversarial Graph Dropout.
EXGC: Bridging Efficiency and Explainability in Graph Condensation.
Invariant Graph Learning for Treatment Effect Estimation from Networked Observational Data.
Graph Anomaly Detection with Bi-level Optimization.
Selected Papers
Honors and Awards
Background
Supervisor: Prof Tat-Seng Chua
Supervisor: Prof Tat-Seng Chua
Supervisor: Prof Tat-Seng Chua; Mentor: Prof Xiangnan He, Prof Liqiang Nie